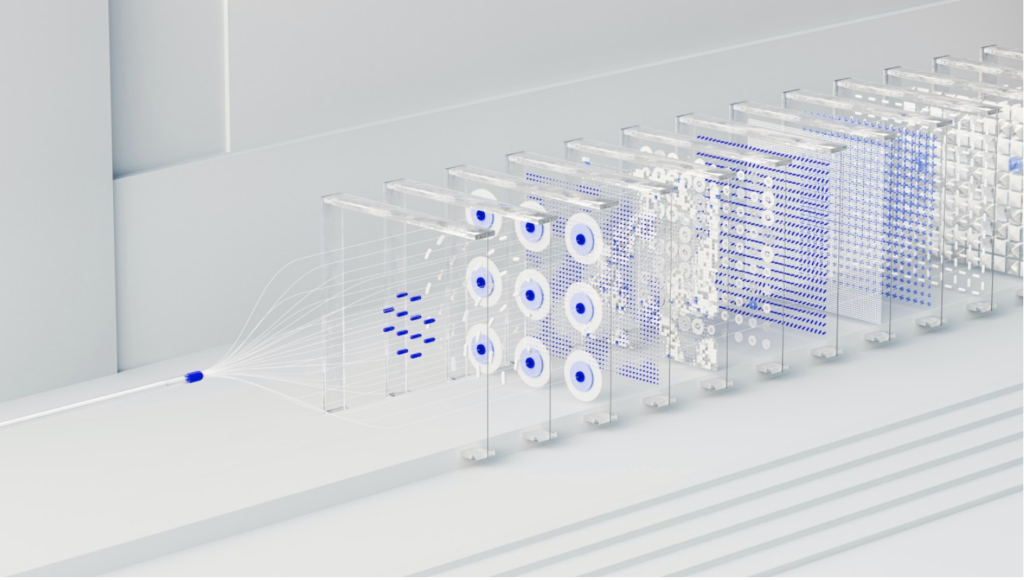
The allure of AI is powerful, drawing in organizations eager for progress and efficiency. Top executives sing its praises at industry events, and the largest companies in the world are at the forefront of utilizing the revolutionary new capabilities in their work. Yet there remains an underlying question beneath the surface for the rest of us: Can AI truly deliver on the lofty expectations, or will teams chasing these benefits be left scrambling to fill in the gaps?
While everyone’s been briefed about AI’s benefits, its associated risks sometimes fly under the radar. While not an exhaustive list, here are three potential blindspots and/or underestimated risks:
- Transparency and Accountability
- Replacing Experts with AI Administrators
- Understand AI Tools and Their Limitations
Transparency and Accountability
In a research setting, issues of replication and reproducibility have been a well-known issue over the past decade. Advances in computational power and advanced tools have only raised the stakes of using complex analytics at scale. In a business setting, the promise of these capabilities comes with a hidden, often unstated implication: businesses are using these analytics to make complex decisions at scale.
This is a key component of how complex tools like AI can inadvertently create unanticipated risks from a business perspective. This is not simply fear-mongering about hallucinations (although negligent use of AI tools without considering this possibility can be extremely harmful). This is an issue that is already known in the field of machine learning; the risk of over-reliance on algorithmic decision-making without proper guardrails in place (or, as discussed later, the proper expertise) can lead to misleading results or, in some cases, even unintended social harm.
Without a deliberate, transparent, and focused effort to develop strong processes around transparency and accountability surrounding these new tools, businesses risk outsourcing decision-making to tools, while shouldering the burden of the consequences. This is not a sustainable model for most companies; one of the most important parts of implementing AI tools into a company will be alignment between stakeholders, executives, and practitioners into the capabilities and boundaries of what a given tool will (and will not) be expected to do.
More importantly, once these capabilities and boundaries are understood, there must be alignment at all levels on how to structure accountability for the decisions made by an AI tool. The potential negative impact of a toxic work environment, where workers are punished (even indirectly, such as requiring engineers to work unreasonable hours) for an AI failure they were never assigned responsibility for, cannot be overstated. As Artificial Intelligence becomes increasingly integrated into the assets (e.g., a website) of a business, the stakes involved with transparent accountability structures only increase alongside it.
Replacing Experts with AI Administrators
It seems natural that implementing these tools will allow an organization to replace highly paid Subject Matter Experts (SMEs) with lower-paid administrators. But it would be a monumental misstep and could have serious consequences for an organization. SMEs are not just overpaid machines spitting out statistical reports or lines of code–they are reservoirs of knowledge, validation, and expertise (as the namesake suggests). While administering prompts and managing processes will be important for utilizing AI in a business environment, SMEs will likely remain a critical part of executing the complex vision of leadership into practice.
The actual role of SMEs, however, may change considerably. Rather than utilizing their expertise in their subject language to generate outputs (e.g., reports, code, etc.), these roles will likely serve a crucial role of translator between leaders, administrators, and tools. Data scientists may become more valuable for their scientific expertise than their data expertise; computer engineers may become more valuable for their engineering capability (interfacing between a human’s vision of a tool and the actual capabilities of a machine to execute it) than for their coding expertise alone. As machine learning approaches machine intelligence, which is ultimately the vision of AI technology, the human element of human capital is also likely to become more valuable.
Before sidelining SMEs for AI and administrators, consider the long-term implications. Utilizing AI technology doesn’t mean sidestepping difficult business questions. Quite the contrary: by implementing machine intelligence in more and more advanced roles, the work of translating between human intelligence and machine intelligence will also increase with corresponding complexity. Validating, learning from, and building human intelligence from machine learning models is already a complex process–subject to extensive debate across all academic fields. This will only continue to grow as an issue as AI continues to become a more normalized part of industry and research.
Ultimately, as industry and research continue to implement more and more complex methods into their work, we cannot neglect to build a corresponding process of validation and learning alongside it–likely of equal or even greater complexity. Failing to take this process seriously can widen the gulf between the humans and machines involved in workflows–increasing the potential risk to both when the two parties are not on the same page.
Understand AI Tools and Their Limitations
A key issue that could fly under the radar is that, as the promise and expectations grow exponentially surrounding new AI tools, stakeholders and leaders quickly risk losing touch with the on-the-ground reality of exactly what new tools are capable of. Without careful consideration of the tools and technologies that actually exist, the rush to implement glossy new technology to ride the marketing wave of an exciting new technology can quickly drown out the voices of those actually in the know about what these technologies can (and can’t) do.
Leaders and stakeholders must understand that now more than ever, the value of actually listening to subject matter experts is at a high point. Marketing materials never have, and never will, sufficiently capture the details of what a given technology is capable of–and the often opaque marketing around new AI tools should raise a caution flag for stakeholders and leaders who are considering investing not only capital but also potential control (for example, the flexibility to move between different proprietary ecosystems), into these new technologies.
Organizations must take seriously the process of piercing the veil of what these tools could do and executing a vision of what these tools can actually do for that organization. This requires investment and planning. It likely requires implementing an AI tool within a narrow scope of an organization’s workflow (e.g., an AI chatbot on a digital site), and more importantly, it requires putting a truly critical lens over whether these small experiments actually provide value. Measuring and validating this performance goes beyond short-term revenue, but (to draw on the previous section) should also rely on expert analysis of where long-term risks lie. Each organization, industry, and organizational culture will have unique problems that should be anticipated prior to a full-scale integration of the technology.
Final Thoughts
Simply put, revolutionary changes cannot be sustained without a revolutionary-scale investment to sustain it. Integrating complex technologies deep into existing workflows requires an equally deep examination of those workflows, in order to ensure transparency for the workers and managers who will ultimately be responsible for that integration. Utilizing AI to pursue higher stakes business goals at scale requires more investment into the human experts who can understand and translate these goals (whether through decades of experience with your organization/industry or through expertise in the affected areas)–not less investment. Finally, any successful business knows that the promise of lofty returns without corresponding levels of risk is no promise at all. AI technology promises high returns. Respect the risk.
Categories