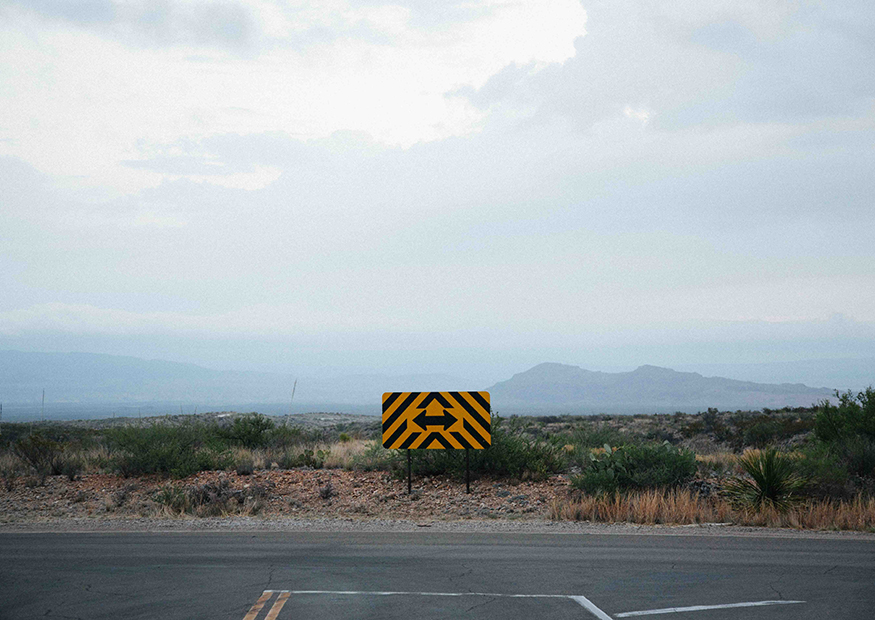
Decision making in business requires, in part, finding certainty in an uncertain world. The importance and beauty of research design derive from finding ways to sensibly navigate what can seem senseless: it helps us understand exactly what we are asking, and what the answers mean for us.
One of the most fundamental things any analyst (or executive managing an analytics team) can do is to embrace the uncertainty surrounding their work and be proactive with identifying and documenting the decisions and assumptions which go into it.
For example, consider a simple question an executive might ask:
“If we implement strategy X now, how will it affect us later?”
There are some very important decisions and assumptions embedded within this statement, each with potentially major business implications.
From a testing perspective, these assumptions and decisions will inform how we approach things like test strategy, statistical methods, and interpretation of results.
While there are many more that could be covered, in this post, we’ll focus primarily on three of these decisions: counterfactuals; decision rules; and the validity-actionability tradeoff. Each is related to the other (and builds off one another), and addressing each can lead to more informed, robust research strategies.
Counterfactuals
One set of decisions involve the design components of a test. In the question above, our executive is interested in “If we implement strategy X.”
The first decision we need to make is to identify our counterfactuals. That is: strategy X as opposed to what? The seasoned analyst, of course, will immediately see this as a question of identifying the individual challengers.
This is important, but there’s actually more to it — there’s an important distinction to be made between a status quo framework or a choice agnostic framework. While we tend to toss around the term “A/B testing,” the simplicity of the term is actually a little deceptive. The A/B terminology actually points more to the latter framework for testing:
“Given a choice between A or B, which should we choose?”
(choice agnostic)
This is a far cry from how we usually test in practice, which is more along the lines of:
“Given that I already have A, should I reject it for B?”
(status quo)
The implications of this decision shouldn’t be underestimated. Businesses spend substantial time, money, and mental effort into developing where they are. An established business with a specific branding strategy, for example, will likely react differently to a bold proposal to change a website’s messaging than a business who is already considering shifting their branding, or a less established business trying to consider their options.
From an analytics perspective, the two approaches have different implications. The status quo approach means an analyst should be more conservative in testing, favoring what is known until proven otherwise.
Here, the testing strategy would focus primarily on whether or not to reject what exists, and the interpretation of results should revolve around whether there is enough evidence to recommend a change, rather than whether one option performs better than the other.
The choice agnostic approach is very different. It means an analyst should primarily favor precision: providing each choice a data-driven analysis on the pros and cons of implementation.
Without explicitly identifying a broader business strategy in a particular test (or broader testing agenda)—in this case, being status quo favoring or choice-agnostic—a testing team risks investing in designs (and potentially full end-to-end tests) that never bear fruit due to foundational conflicts between business strategy and testing strategy.
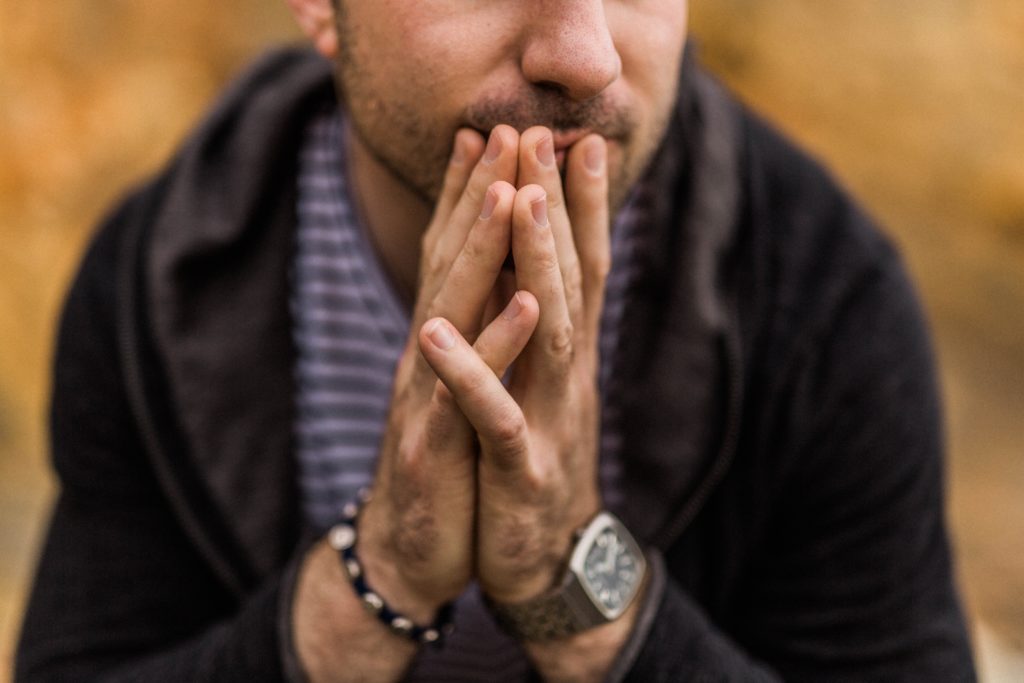
Decision rules
This leads to the second decision we need to make: identifying how we make a decision at all. Returning to our executive’s question above, this revolves around the simple but powerful term: “how?”
Simply put, an effective testing strategy should include careful consideration of how a business approaches decision-making. We can refer to two approaches as choice or probabilistic. Returning to the A/B terminology, we can consider the difference between:
“Should I choose B or should I choose A?”
(choice)
And
“How much should I favor either A or B over the other?”
(probabilistic)
The differences may appear subtle, but these small differences can cascade down the research funnel to impact interpretation or limit the rigor of inferences.
A frequentist significance test, for example, is not designed to provide information on the likely magnitude of lift. Rather, it is designed to provide an interval in which the magnitude is likely to be.
For example, if a frequentist test yields an average lift of 0.04 with a 95% confidence interval of [0.01 , 0.06], the analyst is only able to say that it is 95% likely that this particular experiment’s confidence interval contains the true value (i.e. in 95% of random samples, this confidence interval would contain the true lift), so the average lift is likely to be above zero.
Given this decision rule, the analyst could provide a binary choice to choose the winning variant over the control group. However, a Frequentist confidence test does not provide information about what lifts or values are more or less likely to be real.
Contrast this with a similar lift, using a fully Bayesian approach. Here, we might observe a similar average lift of 0.04, but we also see the full distribution of the data. If the data is normally distributed with a standard deviation of 0.015, for example, we can say that there is a 95% probability that the difference is between 0.01 and 0.06. If the distribution is unimodal, we can provide even more precise estimates of the most likely values: for example, in this case 0.04 is the most likely lift.
If a business prioritizes a probabilistic approach to decision-making, they should weigh this prioritization against investing in the statistical expertise to implement methods which provide a full distribution over possible decisions.
A Bayesian implementation requires careful interpretation and diagnostics, given the distributional assumptions used to generate the posterior distribution. If those words mean nothing to you, then the value placed on making probabilistic decisions should be high enough to warrant investing in the expertise to understand and interpret these methods carefully.
While black-box methods exist, where the assumptions are outsourced to experts external to the business itself, a business risks sacrificing interpretability and transparency by using these. This may be worth the sacrifice, given the potential costs of in-house expertise, but it is a crucial point to consider.
Without specifying whether business decisions are to be made probabilistically or as a binary choice—and, more importantly, whether the decision to do so warrants the requisite investments (or sacrifices)—our business executive from earlier risks investing in tests and arriving out the other side realizing that they need more information they would have been willing to invest in. Conversely, they may have invested into something which they risk interpreting badly.
From an analyst’s perspective, fluency in the languages of probability and hypothesis testing are paramount, since they are often the first point of contact for evaluating testing needs and the information which may (or may not) be possible to glean from a test.
The Validity-Actionability tradeoff
We can think of the third and final decision as the oft-dreaded-but-supremely-important issue of balancing between actionability and validity. For our business executive from above, this is an issue of quantifying the uncertainty around how a test “will” affect the business.
In some ways, this is a culmination of the first two decisions: deciding how to prioritize actionable or valid results comes, in part, from first identifying counterfactuals and settling on decision rules.
A choice-agnostic strategy where decisions are made probabilistically might prioritize actionability: the priority here is to make a choice and move forward. A status quo strategy where decisions are made as choices, however, might prioritize validity: here, it is better to stay where you are in the face of uncertainty until given enough evidence to choose otherwise.
Statistically, bias refers to whether the inferences we make—and the uncertainty we draw around those inferences—reflect some truth about the real world.
Falsely extrapolating from a biased test design, for example, can have implications later on when implementations either do not pan out or, worse, actually turn out to be harmful. A Physicist designing a combustion engine has little to no room for bias, likely erring on the side of caution rather than risking an invalid inference for the sake of actionability.
However, the tradeoff lies in the fact that decisions must be made, given the fact of the world we began with: that the world is uncertain, and there is always the possibility of false extrapolation, imprecise estimates, or faulty samples in a test.
If we relax the fear of invalid inferences, we may be able to better converge around an inference (i.e. a decision): by accepting uncertainty, we are able to embrace our testing and take action even in the face of uncertainty over validity. This does come at a cost, though, and so it requires a business strategy assessing both needs and capabilities with respect to making decisions and moving forward.
A company with very low traffic on their website, for example, might prioritize actionability (higher risk of bias) over validity (lower risk of bias) for the sake of evolution: waiting endless weeks for results to come in from low-traffic sites might render a company unable to adapt, change, and evolve from where it is today.
While this may mean more volatility—for example, greater risk of implementing strategies that end up with a revenue loss—it comes at the gain of real-time learnings through experience. Similarly, a company with extremely high user volume may be able to rapidly test, re-test, and continuously update in ways that prioritize validity slightly higher, since they are able to make better inferences over their user base.
It’s important to understand, though, that both companies could equally make the opposite decisions, given a different set of business incentives. Ultimately, the beauty of test design and research more broadly is the power it places in the hands of those conducting it: to overcome uncertainty, one must first embrace it proactively and realistically.
So, the real lesson here: any analytics professional—be they analysts developing tests or executives presiding over analytics teams—should be unafraid to proactively set realistic expectations about how their business can identify options, make decisions, and take action in the face of uncertainty.