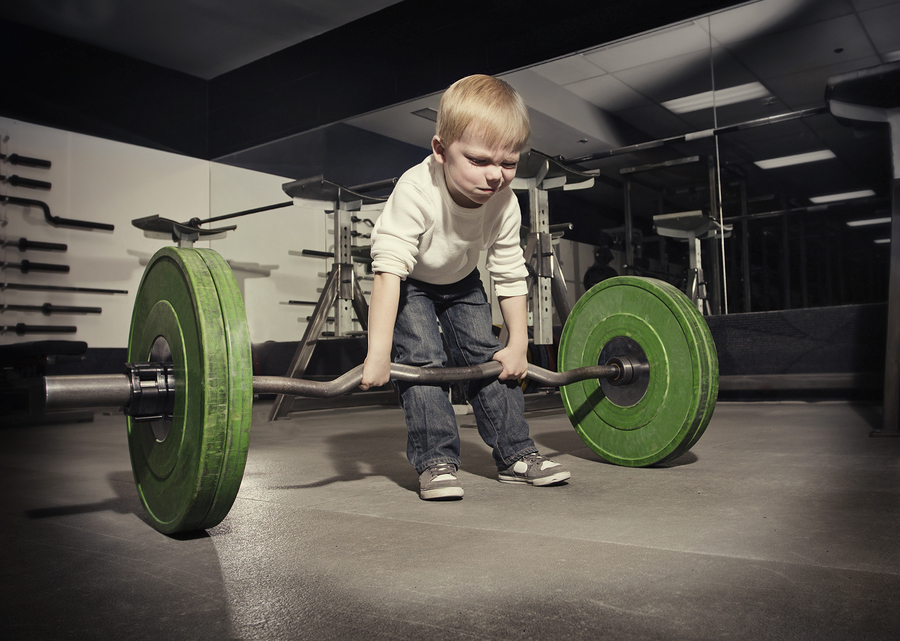
Now you know how to Predetermine Your Test Sample Size and How to Reach Statistical Significance & Power within Your Experimentation Programs. Our final blog concludes the series and will expand your understanding of how to use Minimum Detectable Lift, or Minimum Detectable Effect for optimum test design and results.
Any optimization analyst knows that calculating a test duration prior to launch is a necessary step in reaching the desired level of statistical confidence.
Minimum Detectable Effect (MDE) – a number that represents the relative minimum improvement you seek to detect over the control – is also critical to determining an accurate test duration. (Note: MDE is also known as Minimum Detectable Lift (MDL), and the two terms can be used interchangeably in testing.)
If you’ve ever been confused by this particular input in test design, you’re not alone. As an analyst, I even found it confusing when I first started testing.
Test Design & MDE
Theoretically, an A/B test could last forever without reaching the desired level of statistical significance, or confidence. If the test has little to no impact compared to the control, the test could simply run until someone becomes frustrated and ends it. Similarly, individuals could become impatient with test progression if a website, or a specific set of pages, are not attracting enough visitors to collect a sufficient amount of data. Creating a well-established test design from the start minimizes, if not removes, such frustrations because it relies on data to determine test duration.
The simplest way to think of MDE is the following – it is essentially the smallest possible change in your primary KPI that your experiment can detect with statistical certainty.
Ensuring MDE Success
There are often misconceptions associated with MDE because it is not necessarily a number that can be easily determined without historical testing data or upfront analysis.
For clarification, keep these facts top of mind:
- MDE is not the lift, or conversion increase, that a testing professional wants to see.
- MDE is not a guess.
- MDE is an anticipated lift over the control that can be measured with a degree of certainty.
- MDE is the smallest possible change that would be worth investing the time and money to design the test and implement the change permanently on the site.
The main idea behind MDE is that you will be able to detect a lift down to the specified level at the confidence and significance thresholds you set. For example, if you set a 1% MDE, you will be able to detect a 1%, 3%, or even 10% change in the KPI. It is also important to remember lower MDEs require increased traffic to ensure that a smaller lift is truly valid.
If your organization is establishing a new experimentation program, and historical data is not available, Brooks Bell recommends selecting an MDE from the table below. We have found based on historical analysis, that MDEs vary by page type due to differences in the ability to impact customer behavior at different stages of the online shopping journey.
A 1% increase in Revenue Per Visit from the Cart Page, for example, is going to be much more valuable than a 1% increase in RPV from the Category Page because all revenue goes through the Cart whereas not all revenue passes through a Category Page.
At Brooks Bell, we conduct thousands of online tests for enterprise clients, and collect data from each one. The table below presents website MDE averages by page type.
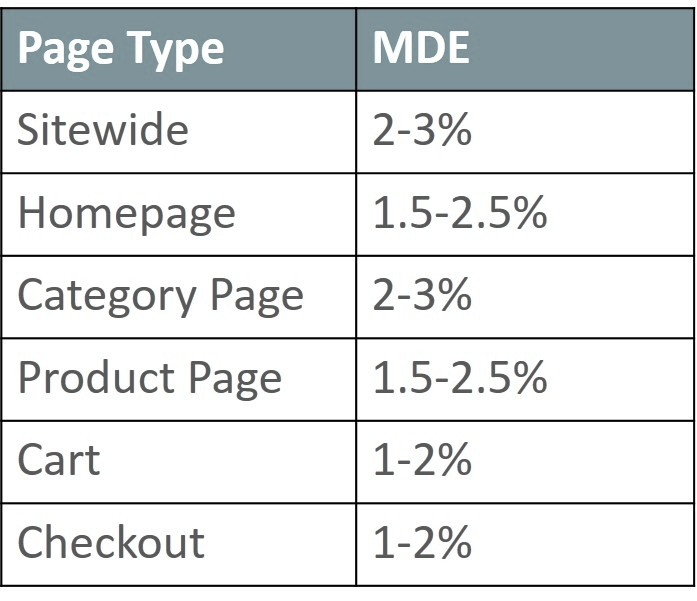
You should use the lowest possible MDE when designing a test, while still running it within an acceptable amount of time. By selecting smaller and more conservative MDE levels, testing programs can optimize the certainty of testing outcomes, learnings, and ultimately ROI.
Brooks Bell helps consumer brands profit through conversion rate optimization, A/B testing, analytics and personalization.
We work with enterprise companies to more effectively leverage their data, create a better understanding of customer segments and build more relevant digital customer experiences. Learn more about our services.