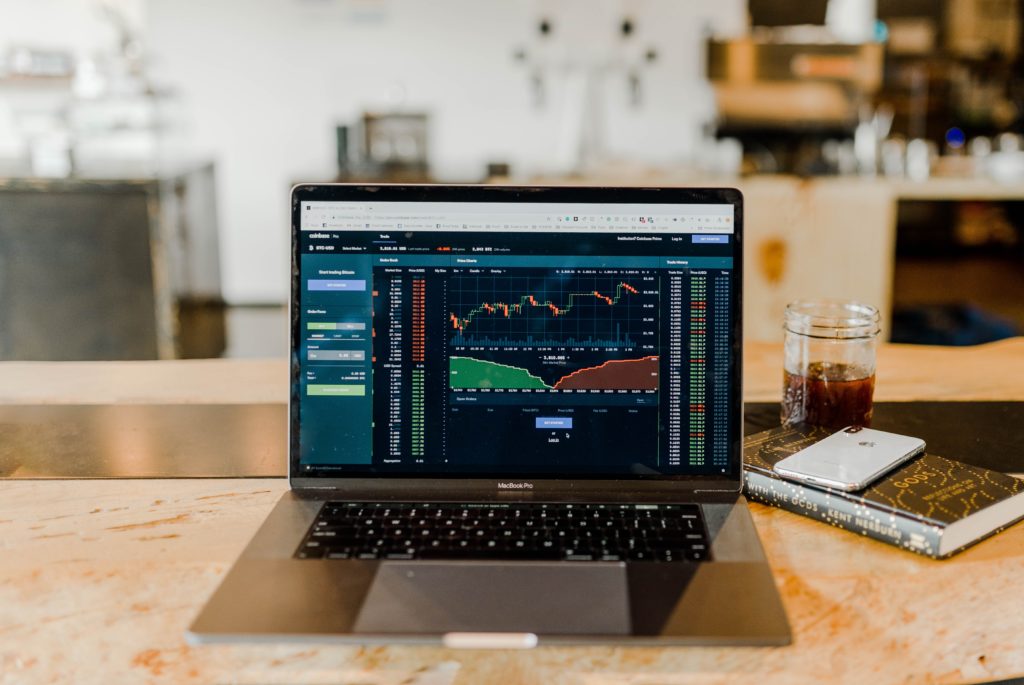
Purpose
In a world of online behavior becoming a heated debate for its ethicality of use, we wanted to share a more altruistic approach in what this data can be used for.
There are undoubtedly thousands of analyses done on predicting COVID hot spots – looking into a wide variety of factors. However, what if these factors could be aggregated into a select few criteria? We asked ourselves the question, “what is impacted by most everything?” Money habits. Spending behavior is likely driven by the same factors that influence someone’s confidence to head out into the world, step into a store or sit in a chair and make a purchase. Moreover, online behavior is made up of much more than just retail – travel and financial industries could be leading indicators of the previously known normality.
- Does a spike in online travel bookings coincide with a COVID spike in a certain location?
- Does an increase in online retail spending suggest that COVID may soon begin to subside in certain areas?
- Does a user banking online suggest they are even too fearful to handle their investments face to face or have more time to take in content?
The point of this is different than most, and was originally not intended to be reactionary – to see what an online retailer might expect based on COVID cases. It was and is intended to be proactive – bringing together these disparate data sources, COVID reporting alongside traffic and conversion trends to attempt to predict where and when COVID is surging or subsiding. As you will see, the following analysis will contain opinions based on the data. These opinions may be controversial to some readers, but they were gleaned from the data and our industry experience.
Analysis
Aggregate Industry View
Using aggregated, anonymized Illuminate(R) data, we’re able to access and trend many different verticals. Joining this with COVID-19 data, both broken down by a state level, we can begin to see interesting trends. We used New Case volume as the indicator of COVID, but deaths were also reviewed and showed similar trends.
Looking at an aggregate conversion view, there are undoubtedly trends specific to travel, retail and financial services verticals.
Of note, there are three major time periods that show substantial increases in COVID cases – 1) the initial onset of the pandemic, 2) late June to early July 2020 and most recently 3) the end of September and into October 2020. These are all based on New Cases. Daily Deaths were also reviewed, and though the scale is much different – similar daily trends are evident. This is documented in many different available reports.
Travel:
The most obvious trend is that Travel took a substantial hit at the beginning of COVID. This has largely returned and stabilized, since mid-June. However, travel is still a bit lower than pre-COVID, and has oddly begun to fall again as we enter October 2020. Sure, there is some seasonality at play, but a closer look reveals that these declines occur at the exact same time as COVID spikes (though, notably not at the same scale). Since a large percentage of travel bookings occur just days before travel, our initial theory was that an increase in bookings would coincide with a COVID increase, perhaps days later.
We do notice that once travel began to gradually increase, there was an increase in confirmed COVID cases toward the tail end of the rise. However, the scale is just not the same, and our data indicates that there were far more quick booking to travel instances. Therefore, in this instance – it would seem that COVID impacts travel more than travel impacts COVID. This is not to say there is not an association, but at the aggregate level – the effect may be muted.
It is worth noting that traffic to travel sites saw a dramatic spike directly before the September 2020 COVID case increase, and declined suddenly thereafter. There is a potential that this was pure consumer interest, but only that – interest while COVID was on the decline.
Retail:
Purchasing behavior has shifted to become more online focused, and many sub-industries (apparel, electronics, etc.) within retail have seen massive gains in online shopping. However, others may have seen declines. Product offering plays a large part in this observed “feast or famine.” An aggregate of retail shows a gradual increase in online purchases that has generally maintained since the beginning months of COVID.
Generally, increases in online purchases have been shortly followed by COVID increases, but that is not always the case. During the first increase, retail conversion increases preceded COVID cases. Traffic was not largely impacted, so this was a pure conversion rate increase that was only seen during the first surge. While the reasoning for this is debatable, we feel a likely explanation is that customers were prepping for quarantine during this early phase, hence why retail increase slightly preceded the COVID spike. As we’ll discuss later, New York was the initial US hot spot, largely contributing to the first surge, and New York has its own special spending behavior.
The second COVID spike was not preceded with an online retail spike or decline. We do know that states began to relax restrictions, but there was not a drastic drop in retail shopping – to suggest that this could predict an approaching wave. The increase in online spending took a sharp increase right after this inflection, and is much more of a reactionary step.
Finally, the last and current increase in COVID is again preceded by an online retail spike (with the same wavelength as seen during the start of COVID). Oddly, this is the inverse of what Travel and Financial online conversion is seeing. Could this be pure seasonality (think Fall and Winter preparation) or is this connected with COVID? In actuality, it’s likely both. There are countless factors we could look at to try to better understand the user’s mindset. It again would appear that retail is more reactionary to COVID than an early predictor. However, it is worth noting that Travel and Retail completely differ in their reaction, which largely makes sense, but when the changes begin occurring at the same time – as in the 1st and 2nd COVID case spikes – they were followed days later by sharp COVID case increases. Is this connected? Perhaps, but nonetheless it’s interesting to note.
Financial:
Despite our expectation to see drastic differences, the use of online financial services has not substantially changed due to COVID and is likely not an indicator of new cases. Specifically, we consider a conversion for financial to range from an account application to content consumption. We would have expected much more account usage and time for exploring online content. However, this just does not seem to be a factor. Many of these items previously required an online presence and even though the market and economy have undoubtedly changed, FSIs are seemingly consistent and least impacted of the major online conversion driving verticals.
Conversion and Traffic Visuals:
Conversions
Traffic
Alternate View: Difference – [COVID New Cases] / [Industry Conversions]
State Level View
There are excellent daily views of COVID cases available, at the state level, so most should understand the key differences in trend. As to why these states differ, well that’s a lengthy debate that we won’t address. However, as has been stated – web behavior could help explain a bit about future COVID case volume. Below are a few plots of highly populated states, and states with either previous or recent COVID case surges.
For the most part, all states have seen similar trends in general web behavior. All took dramatic declines in traffic and conversion at the start of the pandemic, regardless of where their current case volume was. Of course there are outliers, like major urban areas like NYC. However, these cities have much different infrastructure and shopping behavior, even pre-COVID.
It’s notable that of all the states, New York’s web behavior was arguably least affected, but has also seen a relatively slower return to its current state. Looking at specific dates, phase re-openings are easily seen, as is the fall shopping season. It is difficult to assess any COVID impacts for NYC after the initial peak – as case volume is fortunately very low.
Aside from New York and similar states, there are others following different tracks. Texas, California, Florida and really most states have shown dramatic COVID peaks in late July, with declines thereafter. As we take out the potential noise other dis-similar states impart on trends – we do see e-commerce and travel bookings hit their COVID peak right before the COVID case peak, and then begin to decline right after this time. The difficult part is determining at what rate of increase or duration of increase warrants concern? There may be too much involved in each state’s policy to truly understand this, but it is nonetheless very interesting.
North Dakota has been reported as having high COVID cases in recent months. It’s web behavior has also been impacted by this. While many other states have seen e-commerce pick up and maintain starting in April, North Dakota and similar states slowed their e-commerce conversions around June. This, unfortunately was followed by drastic increases in new COVID cases. There are outside factors here, which have been in the news, but states with similar e-commerce patterns have all shown similar COVID case trends.
Could e-commerce affect COVID for specific states? Perhaps – it’s noticeable, but is this because we are biasing ourselves in looking for a trend? I’ll leave that to interpretation.
Though arguably inserting bias – there are states that demonstrate very similar trends, as noted previously. If we look at these, there is an interaction connection between Retail and Travel. As an example. Below is a plot of 13 states with similar trends as Texas, above. The x axis shows Retail conversions while the y axis is COVID case volume. The three potentially confusing lines are a ‘normal’ Travel bookings trend in the middle, with the higher and lower lines representing increased (+1 SD) and decreased (-1 SD) Travel bookings. The plot demonstrates that during periods of high travel and high ecommerce times – new COVID cases are at their maximum. One could counter this point by saying it seeks out a trend, and that’s valid. Regardless, certain states (the majority) do show very similar web behaviors and this behavior has a similar impact on COVID cases.
Takeaway
Can web behavior predict COVID-19 transmission? Our opinion is that, in certain cases, it appears so. There does seem to be a connection between web behavior and new COVID case volume. Specifically, large and quick increases in e-commerce is followed by peak COVID case reports. However, there are many other factors at play, as well. For example – being an extreme urban center will create too much noise in any correlation we may see with the impact of web behavior. Local policy, general public view, etc. will all impact volume. While this was and is known – the thought that this could all boil down to something detectable in web behavior is still a sound theory, but more factors would need to be brought in. It’s a project we’ll continue to investigate, with the goal of bringing these data sources together to better understand the link between online behaviors and COVID so that it can be used for good.
The Data
We’ve released this aggregated and anonymized data to everyone via Illuminate. There, you can see how your traffic and conversion trends compare with top industries or with COVID case reporting. If you have an illuminate account, just log in to access this information. If you don’t have an illuminate account, signup is simple and FREE forever. https://www.brooksbell.com/illuminate/
About the Data:
Information provided for educational purposes only.
Industry trends are an aggregation of similar sites within the specified vertical. Since the purpose of the visual is to display trends – the presented data is adjusted to visually align with COVID-19 data. All daily trends remain consistent with raw data.
Sources:
COVID-19 Data Repository by the Center for Systems Science and Engineering (CSSE) at Johns Hopkins University.
Traffic and conversion data is from illuminate/Brooks Bell.