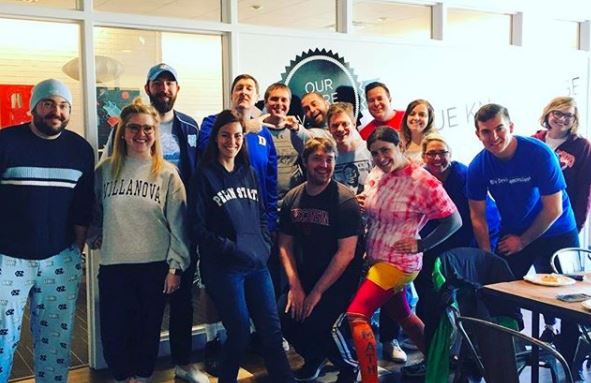
March is here and people around the country are preparing to fill out tournament brackets in anticipation. In addition to taking over the watercooler for a month, the NCAA Tournament famously contributes to a huge dip in productivity. This year it’s projected to cost as much as $6.3 billion as otherwise faithful employees engage in a bit of shirking to catch games. But the month of crazy college basketball isn’t just fun and games. There’s a lot to learn by digging into some basketball data, too.
Basketball fans, of course, try to tease out the key characteristics of each team while building a bracket in an attempt to pick the sure wins and surprise upsets. The odds of getting it all right are astonishingly low. In fact, there’s about a one in 9.2 quintillion chance.
While it’s difficult to predict the winner of the NCAA tournament, it is easier to identify what core characterizes describe a basketball team. For example, a fan can detect the difference between a team that plays up-tempo versus a team that plays more deliberately; studying the statistics would reveal the same inferences about those teams. A similar process can be used to build actionable customer personas as well.
The Struggle of Segmentation
When it comes to segmentation, we want to build a model that can efficiently identify items, in this case basketball teams, that are alike. To accomplish this, a model considers which characteristics best describe each team, then segments the data based on how closely it fits into one of those definitions and how distant it is from the other definitions.
Accomplishing this becomes useful when the segments are meaningful. For example, if we were classifying 2018 data from 64 college basketball teams based on definitions created from data on the last 10 NCAA national champion teams, it might inform styles of play that create a championship team. A marketer, on the other hand, might be able to classify a customer with items in his or her shopping cart based on definitions of price elasticity and make a targeted offer, like a free shipping coupon code, in response.
The Power of Prediction
Since the Brooks Bell headquarters is situated between three historic basketball programs, we decided to look locally to test this idea. Taking data from 2002 to 2018 basketball teams for the University of North Carolina (UNC), Duke University, and North Carolina State University (NCSU), we were able to build a model that could accurately read a set of season-end performance metrics and classify the team. Specifically, we used 15 variables, some of which include: total points, assists, rebounds, free throws and 3-pointer percentage.
Seventeen years of data for three teams gave us 51 teams to classify as either UNC, Duke, or NCSU. The resulting model, based on a Random Forest approach (think of it as the average of many classification-based decision trees), used the statistics to accurately classify about 85 percent of the teams in our sample.
For segmentation purposes, 85 percent accuracy is pretty good, but it’s interesting and instructive to consider why the model misclassified a few of the teams (for example, the 2017-2018 Duke team appears more like a typical UNC team in that they shoot fewer 3-pointers and have a better rebounding margin than traditional Duke teams).
The Personalization Play
As we saw, it’s possible, with a relatively limited set of data, to build a classification scheme that beats 80 percent accuracy. This is great for improving the efficiency of marketing promotions, as well as for segmenting email distribution lists or tailoring product recommendations. And it’s great for identifying iconic teams that generally have definitive playing styles. But sometimes a team that favors small shooting guards picks up two big power forwards in one recruiting class. Or a customer that has three pairs of men’s jeans in his or her cart adds a women’s shirt. As a result, they stop looking like the rest of their true group—according to the model—and start drifting toward a neighboring group.
This is an example of the dilemma that caused misclassification in our model and it’s one of the struggles inherent in personalization. Customers will occasionally act in unexpected ways or share the characteristics of multiple groups. Making segments more granular (i.e. increasing the number of segments) can improve targeting efforts, but only if you have more or better data to feed the model.
Often times there are limitations to models built solely on quantitative information – in these cases, adding additional qualitative information is instructive. In this basketball example, qualitative information would typically come from a fan’s innate understanding of things like coaching tenure, recruiting cohort stability, total team ticket sales, etc.
If classification errors are posing a serious problem to your business, it may be because the data is being pushed beyond its limits. To address lingering questions, a researcher would first conduct in-depth interviews with customers of interest, review videos of customer experiences, read comments and customer service logs. Next, the researcher would use this information to develop an understanding of why segments make sense when they do, and why they don’t when customers fall out of them or are misclassified.
And of course, testing promotions and experiences for segments—like a free shipping offer—is the only sure way to develop a practical and profitable segmentation strategy.
Beat the Buzzer
There’s no better way to close a tight tournament game than with a buzzer-beating three pointer for the win. Here are three points to keep in mind as you enter your own “big dance”:
- What problems are you trying to solve?
Before analysis or modeling begins, it’s important to clearly define the central question to be answered or problem to be solved. - What is the cost and benefit of creating additional experiences?
Simply identifying segments is not enough. Successful segmentation strategies carefully weigh the cost and benefit of serving individual customer groups. Following the ADAMS framework is a great place to start. - What can’t be answered by the data available?
As powerful as modeling can be, it can’t answer every question. Instead of ignoring these lingering unknowns, turn to other methods, including qualitative methods, to continue learning. For many, March Madness may represent a dip in productivity for the year. But it’s also a great reminder of the opportunity segmentation and personalization presents marketers. Even if our brackets are busted, some of the techniques used to characterize basketball teams can lead to a winning customer segmentation strategy.
Reid Bryant, VP of Analytics and Data Science
Reid leads an analytics team responsible for providing the data-driven strategies, insights, and recommendations that guide our optimization efforts. He has 12 years of experience working in analytics, with roles spanning the real estate, finance, and e-commerce sectors, and expertise in data science, data mining, and applied statistics. Reid holds a Master’s in Analytics from Institute of Advanced Analytics at NC State and a BSBA from Kenan-Flagler Business School at UNC-Chapel Hill.