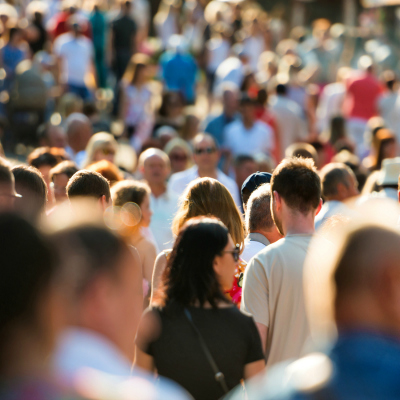
The premise of statistics is simple: “We have a population we want to understand, but we can’t measure everyone. Let’s collect a sample and use statistical inference to make the best decisions we can.”
For a long time, digital testing used simple rules of thumb to answer the next obvious question: “When it comes to determining sample size, how many visitors are enough?” Thankfully, most of us are now using experimental design principles to create stopping rules that lead to more efficient use of site traffic and more trustworthy conclusions.
Figuring out sample size: how we do it
At Brooks Bell, our sample size calculations are grounded in what’s called a power analysis.
A power analysis optimizes the duration of any test so that we can balance false positive errors (tests finding results that aren’t real), false-negative errors (tests failing to find results that are real), and test efficiency (running the test long enough without wasting traffic).
We determine an estimated duration for the test before its launch, taking the testing environment and risk tolerance into consideration. While there are other, fluid methods like sequential testing, a nice byproduct of our methodology is that it facilitates a good testing cadence for our clients and helps with test prioritization.
There are several components of the calculation, and it’s important to understand the working relationship between each component and the resulting test duration.
The components of our sample size calculation are:
- Confidence level
- Power level
- Minimum detectable lift
- Primary success metric (based on type of test being performed)
Confidence level
The confidence level controls what is called type I (false positive) errors, which come from finding a difference that does not actually exist. The industry-standard confidence level is 95 percent. This implies a false positive error rate of 5 percent.
We can vary confidence based on the particulars of the test, but increasing the targeted confidence level would require more visitors to increase test precision. Lowering confidence would allow the test to be completed more quickly but would increase the false positive error rate.
Power level
The power level controls what is called type II (false negative) errors, which come from failing to find a difference that actually exists. The industry-standard power level is 80%. This implies a false-negative error rate of 20%.
We can vary power level based on the specific test, but increasing the targeted power level would require more visitors to increase test precision. Lowering power levels would allow the test to be completed more quickly but would increase the false negative error rate.
Minimum detectable lift
The minimum detectable lift controls how small (precise) of a difference the test is designed to detect. We calculate a specific minimum detectable lift using data collected from the control as the benchmark.
The targeted minimum detectable lift lets us understand the smallest lift we would like to be able to measure; this should be aligned with the smallest lift that could justify using resources to push the winning variation into production. Lower minimum detectable lifts require more precise tests and, therefore, longer run times.
The minimum detectable lift should be sized with respect to the change being made and how similar changes have historically or would theoretically impact performance.
If the change to the page is likely to produce a very small change to the primary success metric, we need to have a very small minimum detectable lift and accept a longer test duration. If the test concepts are on the bolder side, we may expect a larger impact and be willing to accept less precision for a quicker test.
Primary success metric
The last input to the calculation pertains to the primary success metric, which may be binary or continuous. In either case, recent historical data for the control can be used to establish the baseline for which the test hopes to improve upon.
Binary metrics require testing the difference in proportions. Order rate or click-through rate are common examples of binary metrics. If the metric is an action that very few visitors perform, it will take longer to detect any difference.
Continuous metrics require testing the difference between means. Revenue per visitor or average order value are common examples of continuous metrics. For these tests, we need historical data on both the mean of the metric as well as the standard deviation of the metric. The smaller the ratio of mean to standard deviation, the longer the run time. The rationale for this is the wider the spread of the data, the more difficult it will be to detect any difference in performance.
Conclusion: it’s a balancing act
There are always tradeoffs, but at the end of the day reaching confidence is a balancing act of speed and accuracy. However, by understanding each component contributing to the output of sample size calculations, you can contextually plan each test based on your tolerance for errors, desired precision, and time horizon.
Brooks Bell helps top brands profit from A/B testing, through end-to-end testing, personalization, and optimization services. We work with clients to effectively leverage data, creating a better understanding of customer segments and leading to more relevant digital customer experiences while maximizing ROI for optimization programs. Find out more about our services.
Categories